Generative AI has been rapidly gaining popularity and adoption in various industries in recent years, with the global market size expected to reach USD 9.9 billion by 2027, according to a report by ResearchAndMarkets.com.
The report highlights the increasing demand for generative AI in healthcare, automotive, and media and entertainment industries, driven by the technology’s ability to generate realistic images, videos, and text.
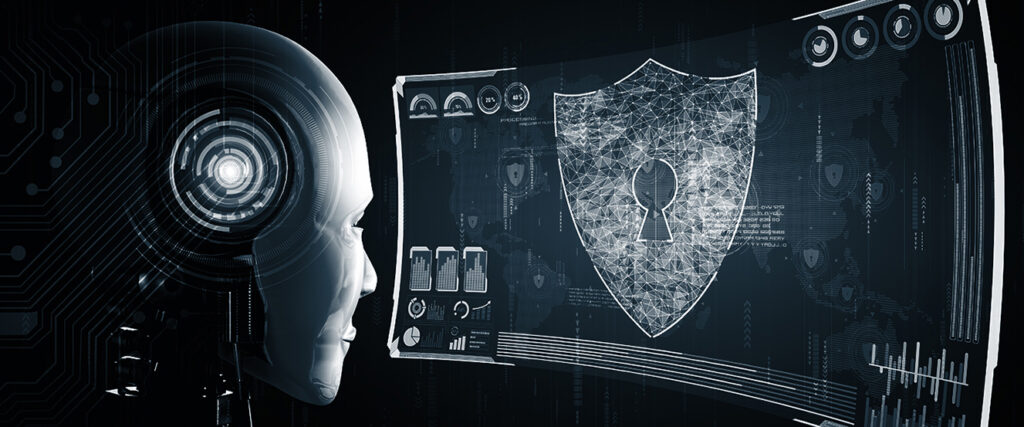
However, securing generative AI models presents significant challenges that need to be addressed. Data security, model security, and usage security are critical in ensuring the privacy and security of generative AI models. According to a report by Accenture, 85% of organizations struggle with securing their AI models, with data security being the most significant challenge.
Policy and compliance also play a crucial role in securing generative AI models. Regulations such as GDPR and CCPA impose strict requirements on the collection, storage, and usage of personal data, including data used for training generative AI models. According to a report by Osterman Research, only 34% of organizations believe they are fully compliant with GDPR requirements.
Generative AI has numerous use cases, including image generation, text generation, and virtual world creation. In the media and entertainment industry, generative AI is used to create realistic special effects and CGI. In the healthcare industry, generative AI is used to generate synthetic data for medical research, reducing the need for collecting sensitive patient data.
The impact of generative AI on synthetic data creation has been significant in various industries, including healthcare, finance, and retail. In healthcare, synthetic data is used to train machine learning models for medical research, drug discovery, and clinical trials, reducing the need for collecting sensitive patient data. In finance, synthetic data is used to test trading algorithms and risk management models, reducing the risk of data breaches and other security threats.
A study by MIT found that using synthetic data for training machine learning models can achieve similar performance to using real-world data, with a significant reduction in costs and time spent on data collection and cleaning. The study highlights the potential benefits of using synthetic data for machine learning, including faster development cycles, reduced bias, and improved data privacy and security.
Despite the challenges, generative AI adoption is expected to continue growing in the coming years due to its potential benefits. In a survey by IBM (Global AI Adoption Index), 64% of enterprises plan to increase their investment in AI by 2022. Moreover, according to a report by MarketsandMarkets, the generative AI market size is expected to grow at a CAGR of 34.2% from 2021 to 2026.
In conclusion, while generative AI offers numerous benefits and opportunities for various industries, securing the data, models, and usage of generative AI models poses significant challenges that need to be addressed. The use of synthetic data is expected to continue growing according to a report by ResearchAndMarkets.com, the global synthetic data market is expected to grow from USD 0.8 billion in 2020 to USD 2.5 billion by 2025. Additionally policy and compliance also play a crucial role in ensuring the privacy and security of generative AI models. However, with the expected growth of the generative AI market and continued investment in AI by enterprises, solutions to these challenges are likely to emerge.